Social determinants of health (SDOH) are the conditions in the environments where people are born, live, learn, work, play, worship, and age that affect a wide range of health, functioning, and quality-of-life outcomes and risks. Understanding data on social determinants of health that can enhance or hinder health, such as income, educational level, employment, language and literacy skills, and access to health care, safe housing, nutritious foods, and physical activity opportunities, can help focus efforts to improve people’s health on a local level.
The medical field has always been data-driven. From patient records to large-scale epidemiological studies, data informs care, research, and policy. In recent times, the emergence of Artificial Intelligence (AI) has unlocked the potential to decode complex data in unprecedented ways. One of the most exciting avenues is the use of AI in medical coding to uncover insights into health equity and social determinants of health.
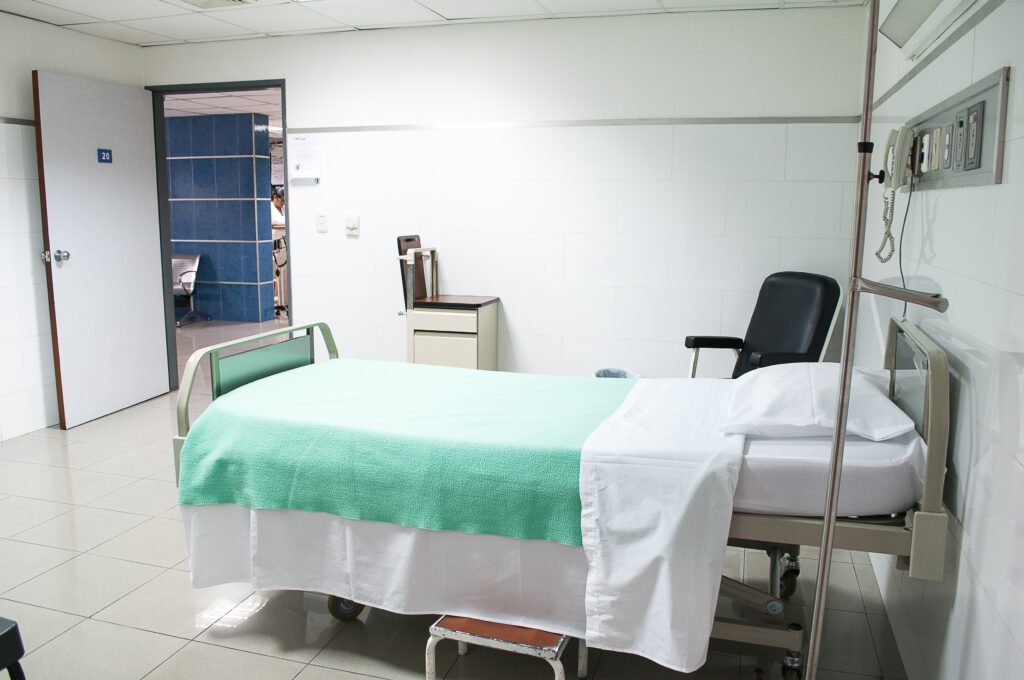
The Power of Medical Coding
Medical coding goes beyond billing and insurance claims. At its core, it’s a robust method of categorizing and recording patient diagnoses, procedures, and care outcomes. This data, when analyzed collectively, can provide a detailed picture of population health, care patterns, and more.
AI’s Role in Deciphering Medical Codes
With the vast amount of medical data generated daily, manual analysis becomes impractical. Enter AI. By processing and analyzing medical codes, AI can:
- Identify Health Disparities: AI can highlight differences in care outcomes across various groups, revealing disparities based on factors like age, gender, ethnicity, and socioeconomic status.
- Determine Social Determinants of Health: By cross-referencing medical data with other datasets (like census data or housing information), AI can uncover social determinants impacting health. These might include factors like education, employment, access to food, and more.
- Predict Health Trends: With its predictive capabilities, AI can foresee potential health crises or outbreaks by analyzing patterns in medical coding data.
Technical Approaches Enhancing AI’s Potential
- Redacting PII: To ensure data privacy and compliance, AI models are trained to redact Personally Identifiable Information (PII) from medical records. This not only protects patient identities but also ensures the data used for analysis is focused purely on health outcomes and trends.
- Training Language Learning Models: Advanced Natural Language Processing (NLP) models can be trained using medical coding data. These models can better understand and interpret medical jargon, leading to more accurate analyses and insights.
- Retrieval Augmentation Using Medical Guidelines: AI can cross-reference medical coding data with established medical guidelines. This approach ensures that the insights derived align with best practices in the medical field.
- Practice Data Integration: By incorporating practice data, AI can gain a holistic view of healthcare delivery. This data can include everything from patient feedback to physician notes, offering a comprehensive picture of healthcare delivery.
Examples of Datasets Containing SDOH Measures:
- American Community Survey (ACS)
- Area Health Resources Files (AHRF)
- Geographic level of data: county
- Publisher: Health Resources & Services Administration (HRSA)
- The AHRF data files include data on health care professions, health facilities, population characteristics, economics, health professions training, hospital use, hospital expenditures, and environment.
- Atlas of Rural and Small-Town America
- Geographic level of data: county
- Publisher: U.S. Department of Agriculture (USDA), Economic Research Service (ERS)
- The Atlas of Rural and Small-Town America provides statistics by broad categories of socioeconomic factors: people, jobs, county classification, income, and veterans.
- Community Resilience Estimates
- Geographic level of data: state, county, census tract
- Publisher: U.S. Census Bureau
- Community resilience is the capacity of individuals and households to absorb, endure, and recover from the health, social, and economic impacts of a disaster such as a hurricane or pandemic. Estimates at the tract and county level are calculated by modeling individual and household characteristics, including poverty, crowding, and unemployment, from the 2019 ACS.
- Crime Data Explorer (CDE)
- Geographic level of data: state, county, city
- Publisher: U.S. Federal Bureau of Investigation (FBI)
- The CDE provides data on violent and property crime incidents.
- Environmental Dataset Gateway (EDG)
- Geographic level of data: county, census tract, census block group
- Publisher: U.S. Environmental Protection Agency (EPA)
- The EDG provides access to EPA’s Open Data resources, including datasets related to air, water, temperature, precipitation, flood, and environmental justice.
- Environmental Justice Index (EJI)
- Geographic level of data: census tract
- Publisher: CDC/ATSDR
- The EJI uses data from the U.S. Census Bureau, the U.S. Environmental Protection Agency, the U.S. Mine Safety and Health Administration, and the U.S. Centers for Disease Control and Prevention to rank the cumulative impacts of environmental injustice on health for every census tract. The EJI ranks each tract on 36 environmental, social, and health factors and groups them into three overarching modules and ten different domains.
- Fatality Analysis Reporting System (FARS)
- Geographic level of data: state, county, point
- Publisher: U.S. Department of Transportation (DOT), National Highway Traffic Safety Administration (NHTSA)
- FARS is a nationwide census providing data regarding motor vehicle traffic crashes with fatal injuries.
- Food Environment Atlas
- Geographic level of data: state, county
- Publisher: U.S. Department of Agriculture (USDA), Economic Research Service (ERS)
- The Atlas provides estimates on three broad categories of food environment factors: food choices (e.g., access and proximity to a grocery store; number of food stores and restaurants), health and well-being (e.g., food insecurity), and community characteristics (e.g., demographic composition; recreation and fitness centers).
- Local Area Transportation Characteristics for Households (LATCH)
- Geographic level of data: census tract
- Publisher: U.S. Department of Transportation (DOT)
- LATCH data provides average weekday household person-miles traveled, person trips, vehicle-miles traveled and vehicle trips at census tract level.
- Local Area Unemployment Statistics (LAUS)
- Geographic level of data: state, county, metro area
- Publisher: U.S. Bureau of Labor Statistics (BLS)
- The LAUS portal provides data on unemployment rates by month and 12-month net changes.
- Location Affordability Index (LAI)
- Geographic level of data: census tract
- Publisher: U.S. Department of Housing and Urban Development (HUD)
- The LAI provides estimates of household housing and transportation costs at the neighborhood-level along with constituent data on the built environment and demographic characteristics.
- National Environmental Public Health Tracking Network
- Geographic level of data: county, census tract
- Publisher: CDC, National Center for Environmental Health (NCEH)
- The Tracking Network is a system of integrated health, exposure, and hazard information and data from a variety of national, state, and city sources.
- Social Determinants of Health Database
- Geographic level of data: county, census tract, ZCTA
- Publisher: Agency for Healthcare Research and Quality (AHRQ)
- The beta data files include data that correspond to five key SDOH domains: social context (e.g., age, race/ethnicity, veteran status), economic context (e.g., income, unemployment rate), education, physical infrastructure (e.g., housing, crime, transportation), and health care context (e.g., health insurance).
- Social Vulnerability Index (SVI)
- Geographic level of data: county, census tract
- Publisher: CDC/ATSDR
- The CDC/ATSDR SVI includes 15 U.S. census variables, including poverty, lack of vehicle access, and crowded housing, that are grouped into four related themes, including socioeconomic status; household composition and disability; minority status and language; and housing type and transportation. Each county and census tract receives a separate ranking for each of the 15 variables, the four themes, as well as an overall SVI ranking.
Extrapolating Levels of Care and Population Health
Using AI to analyze medical coding data can provide insights into:
- Quality of Care: By comparing care outcomes across various facilities or regions, AI can pinpoint areas where the quality of care might be lagging.
- Access to Care: AI can identify regions with insufficient medical facilities or services, guiding policy decisions to improve healthcare access.
- Health Behaviors: Patterns in diagnoses or procedures can shed light on population health behaviors, such as prevalence of smoking, diet, or physical activity.
The Road Ahead
While the potential of AI in using medical coding to assess health equity and social determinants is immense, challenges remain. Data privacy, the need for diverse datasets, and the importance of interdisciplinary collaboration are critical considerations.
Conclusion
As AI continues to make strides in healthcare, its role in using medical coding to uncover insights into health equity cannot be understated. It presents a promising future where data-driven decisions can lead to better care, more equitable health outcomes, and a clearer understanding of the social determinants impacting our health. With the integration of advanced technical approaches, the horizon of what AI can achieve in healthcare seems limitless.